The truth is that we are just at the beginning of the Artificial Intelligent (AI) revolution. The capabilities of AI are just now starting to show hints of what the future holds. For instance, cars are using large complex neural network models to not only understand their environment, but to also steer and control themselves. For any application there must be training data to create useful networks. The size of both the training and inference operations are growing rapidly as useful real-world data is incorporated into models. Let’s look at the growth of models over recent years to understand how this drives the needs for processing power for training and inference.
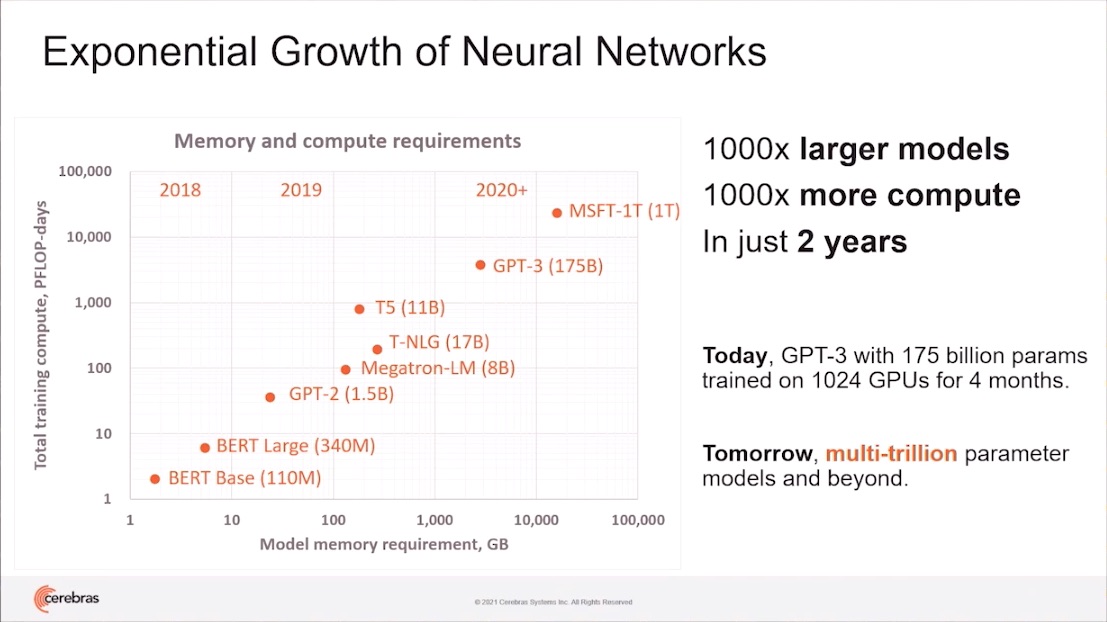
In a presentation at the Ansys 2021 Ideas Digital Forum, the VP of Engineering at Cerebras, Dhiraj Mallik, provided some insight into the growth of neural network models. In the last two years model size has grown 1000X, from BERT Base (110 MB) to GPT-3 (175 GB). And in the offing, there is the MSFT-1T model, with a size of 1 TB. The GPT-3 model – which is an interesting topic of its own – was trained with conventional hardware using 1024 GPUs for 4 months. It’s a natural language processing (NLP) model that uses most of the text data on the internet and other sources. It was developed by Open AI, and is now the basis for the OpenAI Codex, which is an application that can write useful programming code in several languages from plain language instructions from users. GPT-3 can be used to write short articles that a majority of readers cannot tell were written by an AI program.
As you can see above, running 1024 GPUs for 4 months is not feasible. In his talk titled “Delivering Unprecedented AP Acceleration: Beyond Moore’s Law” Dhiraj makes the point that the advances needed to support this level of semiconductor growth go far and away beyond what we have been used to seeing with Moore’s Law. In response to this perceived market need, Cerebras released their WSE-1, wafer scale AI engine in 2019 – 56 times larger than any chip ever produced. A year and half later they announced the WSE-2, again the largest chip every built with:
- 6 trillion transistors
- 850,000 optimized AI cores
- 40 GB RAM
- 20 petabytes/s memory bandwidth
- 220 petabytes fabric bandwidth
- Built with TSMC’s N7 process
- A wafer contains 84 dies, each 550 mm2.
The CS-2 system that encapsulates the WSE-2 can fit AI models with 120 trillion parameters. What is even more impressive is that CS-2 systems can be built into 192-unit clusters to provide near linear performance gains. Cerebras has developed a memory subsystem that disaggregates memory and computation to provide better scaling and improved throughput for extremely large models. Cerebras has also developed optimizations for sparsity in training sets, which saves time and power.
Dhiraj’s presentation goes into more detail about their capabilities, especially in the area of scaling efficiently with larger models to maintain throughput and capacity. From a semiconductor perspective it is also interesting to see how Cerebras analyzed the IR drop, electromigration, and ESD signoff on a design that is 2 orders of magnitude bigger than anything else ever attempted by the semiconductor industry. Dhiraj talks about how at each level of the design – tile, block, and full wafer – Cerebras used Ansys RedHawk-SC across multiple CPUs for static and dynamic IR drop signoff. RedHawk-SC was also used for power electromigration and signal electromigration checks. Similarly, they used Ansys Pathfinder for ESD resistance and current density checks.
With a piece of silicon this large at 7nm, the tool decisions are literally “make or break”. Building silicon this disruptive requires a lot of very well considered choices in the development process, and unparalleled capacity is of course a primary concern. Yet, as Dhiraj’s presentation clearly shows, CS-2’s level of increased processing power is necessary to manage the rate of growth we are seeing in AI/ML models. Doubtless we will see innovations that are beyond our imagination today in the field of AI. Just as the web and cloud have altered technology and even society, we can expect the development of new AI technology to change our world in dramatic ways. If you are interested in learning more about the Cerebras silicon, take a look at Dhiraj’s presentation on Ansys IDEAS Digital Forum at www.ansys.com/ideas.
Also Read
SeaScape: EDA Platform for a Distributed Future
Ansys Talks About HFSS EM Solver Breakthroughs
Ansys IDEAS Digital Forum 2021 Offers an Expanded Scope on the Future of Electronic Design
Share this post via:
Comments
There are no comments yet.
You must register or log in to view/post comments.