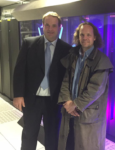
Professor Chris Eliasmith (right side) is co-CEO and President of Applied Brain Research Inc. Chris is also the co-inventor of the Neural Engineering Framework (NEF), the Nengo neural development environment, and the Semantic Pointer Architecture, all of which are dedicated to leveraging our understanding of the brain to advance AI efficiency and scale. His team has developed Spaun, the world’s largest functional brain simulation. He won the prestigious 2015 NSERC Polanyi Award for this research. Chris has published two books, over 120 journal articles and patents, and holds the Canada Research Chair in Theoretical Neuroscience. He is jointly appointed in the Philosophy, Systems Design Engineering faculties, as well being cross-appointed to Computer Science. Chris has a Bacon-Erdos number of 8.
Peter Suma (left) is a co-CEO of Applied Brain Research Inc. Prior to ABR, Peter led start-ups in robotics and financial services as well as managed two seed venture capital funds. Peter holds degrees in systems engineering, science, law and business.
What is ABR’s vision?
ABR’s vision is to empower the world’s devices with intelligent, concept-level conversations and decision-making abilities using our innovative Time Series Processor (TSP – https://appliedbrainresearch.com/products/tsp/) chips.
Whether it’s enabling full voice and language processing on a small, low-power chip for consumer electronics and automotive applications, processing radar signals faster and for less power, bringing cloud-sized AI signal processing on to devices, or integrating situational awareness AI to give robots the ability to understand and respond to complex commands to interact with people in a natural and intuitive way, our TSP chip family is poised to revolutionize the way devices sense and communicate.
ABR has been delivering advanced AI R&D projects since 2012 to clients including the US DoD, Intel, BMW, Google, Sony and BP. Some examples of our work include, developing the world’s largest functional brain simulation, building autonomous drone controllers for the US Air Force, and building small, powerful voice control systems for cars, appliances and IoT devices. Our TSP chips are our latest innovation as we work to fit more and better AI models into devices to give devices better artificial ‘brains’.
How did ABR begin?
ABR was founded out of Dr. Chris Eliasmith’s lab at the Centre for Theoretical Neuroscience at the University of Waterloo. Applied Brain Research Inc. (ABR) is now a leading brain-inspired AI engineering firm. Our AI engineers and neuroscientists develop technologies to improve AI inspired by work in AI and brain research at the lab.
You mentioned you have some recent developments to share. What are they?
We are very excited to announce that ABR has been admitted to the ventureLab and Silicon Catalyst Incubator programs to support the development of our new Time Series Processor (TSP) family of edge AI chips, which allow cloud-sized speech and signal AI models to run at the edge at low cost, power, and latency. We will be exhibiting at CES in the Canada-Ontario Booth in the Venetian Expo Hall D at booth number 55429 from Jan 5th to Jan 8th, 2023, in Las Vegas. ABR is also a CES Innovation Awards Honoree (https://appliedbrainresearch.com/press/2022-11-21-ces-innovation-awards/) this year.
Tell us about these new chips you are building?
Most electronic devices already do, or will soon have to, utilize AI to keep pace with the smart features in their markets. More powerful AI networks are larger AI networks. Today’s edge processors are too small to run large enough AI models to deliver the latest possible features, and CPUs and GPUs are too expensive for many electronic devices. Cloud AI is also expensive, and for many products connections cannot be guaranteed to be accessible and are often not configured correctly by the customer.
What device makers need is a small, inexpensive, low-power chip that can run large AI models to enable the products to lead their respective markets. A very efficient, economical, and low-power way to achieve this is to compress large AI models and design a computer chip that runs these compressed models.
ABR has done exactly this with a new patented AI time-series compression algorithm called the Legendre Memory Unit or LMU. With this compression algorithm we have developed a family of small but very powerful time series processing AI processors that run speech, language and signal inference AI models in devices that previously would have required a cloud server.
This enables more powerful and smarter devices with low power consumption. Batteries last longer, devices converse in full natural language sentences, and sensors process more events with greater accuracy. ABR is enabling a new generation of intelligent devices with our revolutionary low-power, low-cost and low-latency powerful AI Time Series Processor (TSP) for AI speech, language and signal processing.
What are the chips in the ABR TSP family?
There are currently two chips in the ABR TSP family. The Chat-Chip TSP and the Signal-TSP.
The ABR Chat-Chip TSP is the world’s first all-in-one full voice dialog interface, low-power chip. Low-cost and low-power speech chips until now have been limited to keyword-spotting AI models which are limited to understanding 50 or so words. These chips deliver those oh-so-frustrating speech interfaces in cars, toys and other speech-enabled, sometimes-disconnected, low-BOM-cost devices. ABR’s Chat- Chip TSP replaces those chips for the same cost with a full natural language experience. Dramatically upgrading the customer’s experience.
The ABR Chat-Chip enables a full natural language voice assistant in one chip, including noise filtering, speech recognition (ASR), natural language processing (NLP), dialog management, and text-to-speech (TTS) AI. The ABR Chat-Chip TSP can run cloud-sized speech and language AI models in one chip, consuming less than 50 milli-watts of power. This combination of low-cost, low-power and large speech and language AI model processing means the ABR Chat-Chip TSP brings full Alexa-like natural language dialog to all devices including devices that, until now, could never have implemented full language dialog systems due to cost, latency and model size limitations when using existing chips.
Cameras, appliances, wearables, hearables, robots, and cars can all carry on complex, real-time, full language dialog with their users. People can hear better with larger de-noising and attention-focusing AI models in earpieces. People can interact with devices, more privately, instantly, and more hygienically without touching buttons. The many robots in our lives now and the near future can interact verbally without a cloud connection. Devices can also explain to users how to use them, offer verbal troubleshooting, deliver their user manuals verbally, offer hygienic, touchless interfaces, handsfree operation, and market their features to consumers. All of this without needing an internet connection, but able to take advantage of one if present. Voice interfaces delivered locally are more private, as they do not send sound recordings to the cloud, eliminating the risk of leaking background noise and emotional context. As well, local dialog processing is faster, without the latency of a cloud network. Local dialog processing reduces device makers’ costs per device and in the cloud, by removing large portions of the cloud processing needed for voice interfaces and performing the local processing at up to 10x less in-device processor cost.
The ABR Signal-TSP performs AI signal pattern and anomaly detection by running larger AI models, faster and for less power than existing CPUs and GPUs. In a market where larger AI models are typically much more accurate AI models, device makers need inexpensive, low-power, large AI model processors to make their devices smarter than the competition’s. ABR’s Time Series Processors (TSPs) cost just few dollars but run large AI models that otherwise would require a full CPU or GPU costing between $30 to $200 USD to execute the same workload in real-time. ABR’s Signal TSP typically reduces power consumption by 100x, latency by 10x and cost by 10x over functionally equivalent CPUs or GPUs.
How are the TSP chips programmed?
ABR supports the TSP chips with an API and an AI hardware deployment SaaS platform called NengoEdge (edge.nengo.ai). AI models can be imported from TensorFlow and then optimized for deployment to the TSP and other chips using NengoEdge. With NengoEdge you can pick a network, set various hardware-aware optimizations, and then have NengoEdge train and optimize the network using hardware specific optimizations, including quantization and utilization of any available AI acceleration features, such as the LMU fabric if a TSP is targeted. The result is an optimal packing of the AI network onto the targeted chips to deliver the fastest, lowest-power and most economical solution for delivering the chosen network onto the target hardware. All without buying each chip to test or learning the details of each chip. Users can see the TSP shine on all time series workloads, for example for voice assistants or radar processing AI systems.
Can you tell us more about your LMU compression algorithm?
The Legendre Memory Unit (LMU) was engineered by emulating the algorithm used by time cells in the human brain and specifically how time cells are so efficient at learning and identifying event sequences. The LMU makes the ABR TSP’s large gains in efficiency, performance and cost possible for inferencing all time series and sequence-based AI models. We patented the LMU worldwide in 2019 and announced it NeurIPS in December 2019. We then published the software versions of the LMU on our website and GitHub in 2020. There are many papers now published using the LMU and achieving state of the art results on time series workloads by other groups. We have many clients who have licensed the LMU software running on CPUs, GPUs or MCUs for signal and speech processing in devices such as wearables, medical devices and drone controllers. Many of those are now waiting to move to a TSP chip to extend their battery life and support even larger models at lower power, cost and latency levels.
When will the TSP chips be available?
We are working to have first silicon TSP chips for both the Chat-Chip and Signal design available by Q1 2024. We are signing pre-orders and design LOI’s now. Contact Peter Suma, co-CEO of ABR at peter.suma@appliedbrainresearch.com or on 1-416-505-8973 to learn how we can super charge your devices to be the smartest in their class.
Also Read:
CEO Interview: Ron Black of Codasip
CEO Interview: Aleksandr Timofeev of POLYN Technology
CEO Interview: Coby Hanoch of Weebit Nano
CEO Interview: Jan Peter Berns from Hyperstone
Share this post via:
Comments
There are no comments yet.
You must register or log in to view/post comments.