Key Takeaways
- Synopsys User Group (SNUG) Conference highlighted the evolution of Synopsys and the EDA industry, showcasing continued innovation and leadership.
- Sassine Ghazi emphasized the need to 're-engineer engineering' for AI-centric silicon design, shifting the traditional process to prioritize software workloads.
- Microsoft's Satya Nadella discussed the accelerating complexity in hardware and software design, likening it to 'Moore’s Law on hyperdrive'.
Hot on the heels of DVConUS 2025, the 35th annual Synopsys User Group (SNUG) Conference made its mark as a defining moment in the evolution of Synopsys—and the broader electronic design automation (EDA) industry. This year’s milestone event not only underscored Synopsys’ continued innovation but also affirmed the vision and direction of its new leadership.
The conference opened with a keynote address from Synopsys President and CEO Sassine Ghazi, setting the tone for two packed days of technical exploration. Attendees had access to 10 parallel tracks covering everything from analog and digital design to system-level innovations, with real-world case studies across IP, SoCs, and emerging system-of-systems built on cutting-edge multi-die architectures.
Highlights included a Fireside Chat, a thought-provoking panel discussion, and another keynote now delivered by Richard Ho, Head of Hardware, OpenAI, titled “Scaling Compute for the Age of Intelligence,” each drawing a full house of users, Synopsys staff, media, and industry influencers.
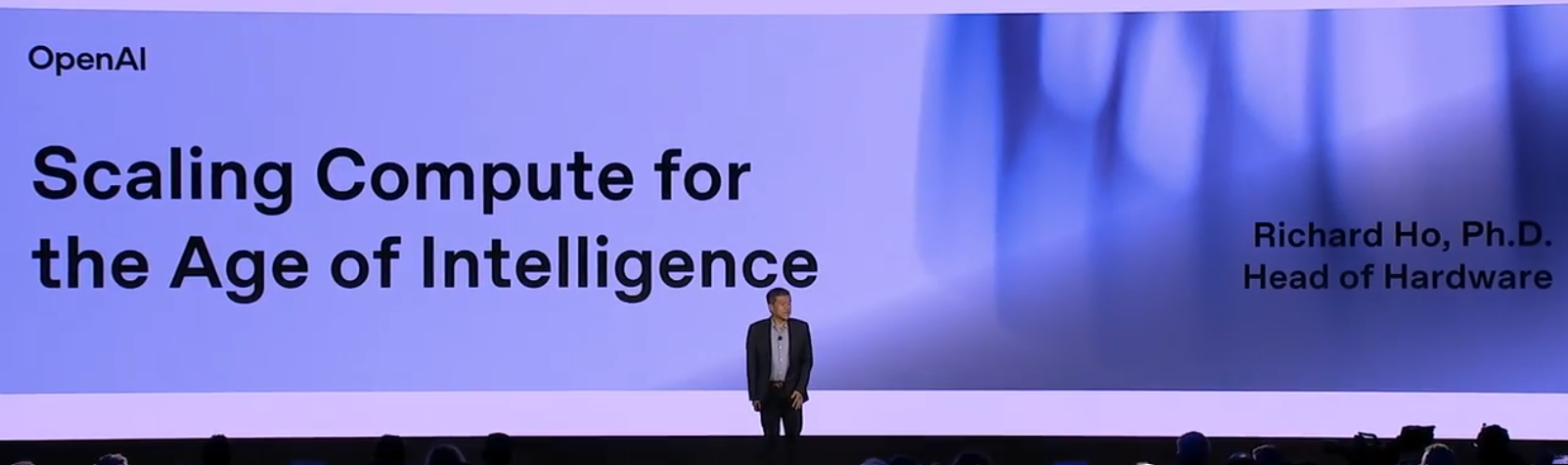
The exhibit hall buzzed with energy as users connected directly with Synopsys engineers and executives, exchanging insights and exploring the latest tools and solutions.
Keynote by Sassine Ghazi, Synopsys’ President and CEO: Re-engineering Engineering
Sassine set the stage by highlighting the arrival of a new era—one defined by pervasive intelligence. This shift promises to deliver unprecedented innovation and disruption, fueled by a surge of AI-powered products built on advanced AI silicon. To realize this future, there is a need for highly efficient silicon, which in turn demands a rethinking of how to engineer systems. In Sassine’s words, we are entering a time when we must “re-engineer engineering itself.”
Designing AI-centric silicon must turn the traditional design process on its head. Instead of developing computing hardware in isolation and testing it against software workload only during the validation stage, the new approach puts the software workload on the driving seat, using it from the outset to shape the processing hardware architecture.
This new paradigm requires deep collaboration across the ecosystem, including partnerships with established semiconductor leaders and cutting-edge startups alike. Underscoring the importance of such collaboration, Sassine welcomed Microsoft CEO Satya Nadella to join him remotely on stage to share his vision for the road ahead. Nadella described the moment as “Moore’s Law on hyperdrive,” with scaling laws accelerating across multiple S-curves. Hardware and software complexities are now growing in tandem aiming at delivering super-fast performance while consuming less energy at reduced unit costs.
He also outlined the critical and evolving role of AI in the design process, describing it as a journey through three distinct phases. In the beginning, engineers asked questions and executed tasks manually. Today, we’re in the second phase where engineers issue instructions and AI handles the execution, though human oversight remains crucial. In the next phase, AI will take on a more autonomous role, making design decisions to generate high-quality, well-optimized products. But even as abstraction levels rise, the role of the engineer will not disappear. A deep understanding of systems will remain essential to guiding and validating AI-driven products.
One of the most significant developments over the past year, Nadella noted, has been the growing need for AI models to reason—not just execute. While many pre-trained models are quite capable, true progress lies in teaching these models to reason effectively for specific tasks. In silicon design, that means enabling AI to make smart trade-offs to optimize power, performance, and area. It’s not just about what the model knows—it’s about how it reasons through complexity to produce better engineering solutions.
Sassine went on to address the escalating complexity of chip design, emphasizing the challenge of building systems with hundreds of billions—or even trillions—of transistors using angstrom-scale process technologies. These designs are increasingly implemented across multiple dies integrated into a single package, while compressing development timelines—from the traditional 18-month tapeout cycle down to 16, 12 months, or even less—to deliver highly customized silicon for next-generation intelligent systems.
Sassine explained that tackling this complexity demands technological evolution across six key dimensions of the design process:
- 3D IC Packaging – Leveraging multi-die systems built on different process technologies and sourced from multiple foundries is essential for efficiently mapping trillions of transistors.
- Innovative IP Interfaces – High-performance, power-optimized communication between chiplets in multi-die assemblies is critical to meeting system-level targets.
- Advanced Process Nodes – Progressing into the angstrom era requires entirely new approaches to scaling and integration.
- Next-Generation Verification and Validation – Cutting-edge techniques are needed to enable effective hardware/software co-design and support shift-left methodologies.
- Silicon Lifecycle Management (SLM) – With schedules tightening, verification must extend from pre-silicon to post-silicon and continue into in-field testing to ensure ongoing quality and performance.
- Holistic EDA Methodologies – Tools must now encompass the full stack—from front-end to back-end, assembly to packaging—bridging both the abstract and physical domains, and extending beyond electronics to account for thermal, mechanical, structural, and fluidic challenges.
Sassine then turned to how Synopsys has been embedding AI into its tool suite to address these growing demands and transform the design process.
The journey began in 2017 with Synopsys.ai through the pioneering use of reinforcement learning in physical implementation, introducing DSO.ai to work collaboratively with Fusion Compiler. The goal was to optimize an enormous design space with countless inputs to deliver the best possible PPA—power, performance, and area—in the shortest time.
That was followed by the data continuum—a framework that connects insights across the design and manufacturing lifecycle. Design.da, Fab.da, and Silicon.da, used analytics to inform what happens at each successive stage of the flow. In 2018 a working prototype delivered fantastic results on real customer designs, but it was also met with a mix of skepticism and confusion. Customers weren’t sure how to integrate this technology into workflows that had been optimized over decades. Engineers being often skeptical of new methods do not embrace changes easily. But today, Synopsys.ai has become essential for achieving the level of productivity and quality needed to keep pace with growing complexity.
Generative AI opened up new opportunities for innovation at Synopsys. Generative AI includes two parts: assistive and creative. The assistive side encompasses a co-development with Microsoft to introduce Copilot-style capabilities—workflow assistants, knowledge assistants, debug assistants. These tools help both junior and senior engineers ramp up faster and interact with Synopsys software in a more modern, efficient way. The creative side supports tasks like RTL generation, testbench creation, documentation, and test assertions. Here is where Copilot not only assists but also creates. The productivity gains are game-changing, compressing tasks that once took days into just minutes.
As AI continues to evolve, so too is the design workflow. The rise of agentic AI has sparked the vision of agent engineers—AI collaborators that will alongside human engineers to manage complexity and reshape the design flow itself. This is where Synopsys is investing in partnerships with leaders like Microsoft, NVIDIA, and others to develop domain-specific agents tailored for the semiconductor industry.
At this stage, Sassine summarized the adoption of AI from the beginning into the future via a roadmap charting on the X-axis the evolution from copilot to autopilot and on the Y-axis the cumulative capabilities—from generative AI to agentic AI—layered step by step. See figure 2.
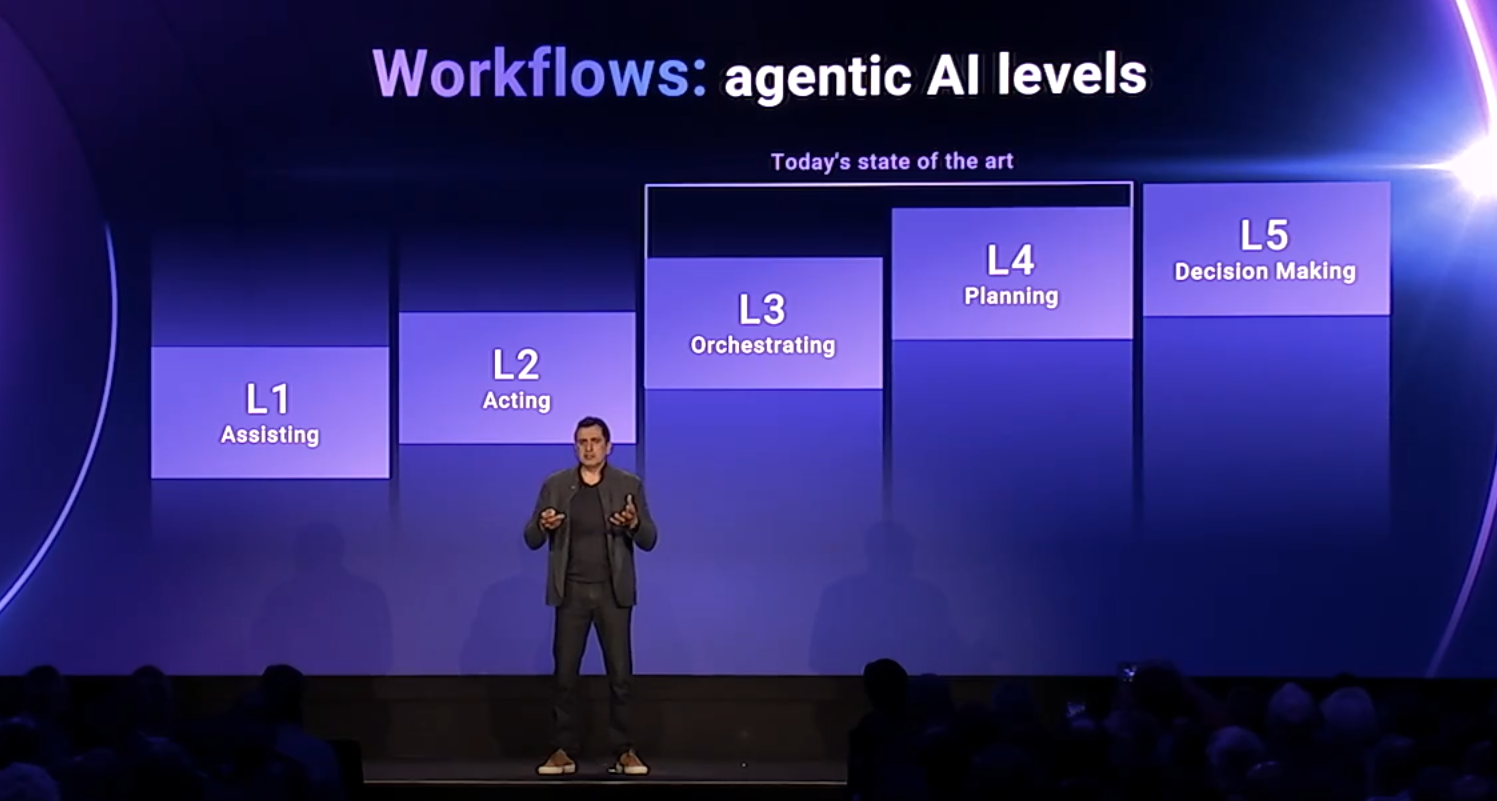
The initial focus was on assistance. Over the past few years, copilot-style capabilities were embedded in each of Synopys tools with copilots powered by domain-specialized LLMs, trained specifically for their respective tasks—whether it’s synthesis, simulation, or verification. This foundational step is to be followed by the adoption of action introduced by agents purpose-built for specific tasks. For example, an agent for RTL generation, another for testbench creation, or one focused on generating test assertions. These task-specific agents will continually improve as they learn from real-world designs and the unique environments of each customer.
As the technology matures, we move into the orchestration phase: coordinating multiple agents to work together seamlessly. From there, we progress to dynamic, adaptive learning—where agents begin optimizing themselves based on unique workflow and design context.
Initially, agents will operate within existing workflows. But as orchestration and planning capabilities advance, the workflows themselves will begin to evolve. The ultimately goal is to build a framework where agentic systems can autonomously act and make decisions—on a block, a subsystem, or even an entire chip—driving toward a future of intelligent, self-directed design.
To conclude, Sassine drew a parallel to the levels of autonomous driving—from L1 to L5—where the progression goes from human-monitored systems to fully autonomous vehicles. In the early stages (L1-L2), the driver remains in control, while higher levels shift responsibility to the system itself. The adoption of AI agents in engineering is going to follow a similar framework—a path from today’s assistive copilots to future autonomous, multi-agent design systems. See figure 3.
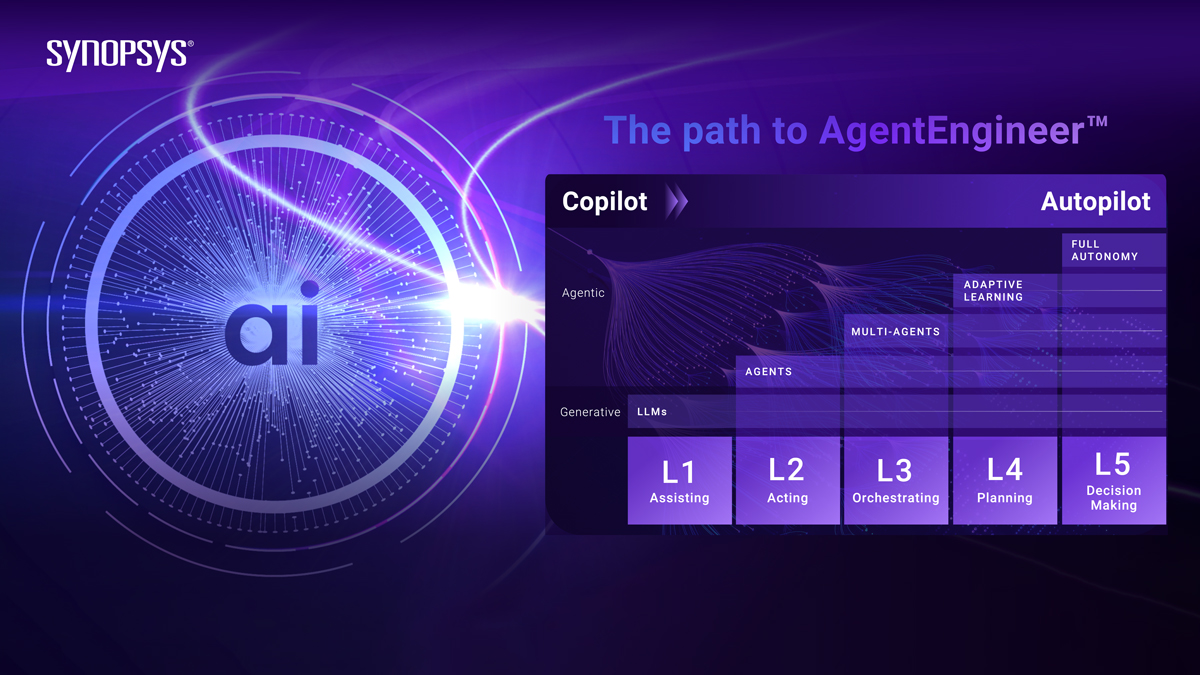
At Level 1, todays’ copilots are AI assistants embedded within Synopsys tools that support engineers with tasks like file creation or code generation using large language models. They help, but they don’t act on their own.
Level 2 introduces task-specific action. Here, agents can begin executing defined tasks within a controlled scope. For instance, an agent could fix a link error, resolve a DRC violation, or make other focused adjustments—with the human engineer still actively involved in oversight and decision-making.
At Level 3, the realm of multi-agent orchestration begins. This is where agents collaborate across domains to solve more complex challenges. As examples, signal integrity or timing closure issues that span over multiple parts of the flow require coordination among several specialized agents to achieve resolution.
Level 4 adds planning and adaptive learning. At this stage, agent systems begin to assess the quality of their own outputs, refine the flow, and adapt over time. The workflow itself begins to evolve—moving beyond the static, predefined flows of earlier levels.
Finally, Level 5 is what we consider true autopilot. Here, a fully autonomous multi-agent system can reason, plan, and act independently across the entire design process. It has the intelligence and decision-making capability to achieve high-level goals with minimal human input.
Today, Synopsys is actively operating at Levels 1 and 2, with a growing number of real-world engagements across customers. These systems are assisting and acting in limited scopes, and are continuously enhanced. Just like in autonomous driving, reaching L3 or L4 doesn’t mean abandonment of L1 or L2. Each level builds upon the last—constantly evolving and coexisting as the technology matures.
In wrapping up, Sassine returned to two key ideas: the need to re-engineer engineering, and the rise of agent engineers working in tandem with human engineers. Together, they will drive the workflow transformation required to meet the scale, complexity, and speed of what lies ahead.
Also Read:
Synopsys Webinar: The Importance of Security in Multi-Die Designs – Navigating the Complex Landscape
Synopsys Executive Forum: Driving Silicon and Systems Engineering Innovation
Evolution of Memory Test and Repair: From Silicon Design to AI-Driven Architectures
Share this post via:
Comments
There are no comments yet.
You must register or log in to view/post comments.