Ironwood is our most powerful, capable and energy efficient TPU yet, designed to power thinking, inferential AI models at scale.
Amin Vahdat
VP/GM, ML, Systems & Cloud AI
Today at Google Cloud Next 25, we’re introducing Ironwood, our seventh-generation Tensor Processing Unit (TPU) — our most performant and scalable custom AI accelerator to date, and the first designed specifically for inference. For more than a decade, TPUs have powered Google’s most demanding AI training and serving workloads, and have enabled our Cloud customers to do the same. Ironwood is our most powerful, capable and energy efficient TPU yet. And it's purpose-built to power thinking, inferential AI models at scale.
Ironwood represents a significant shift in the development of AI and the infrastructure that powers its progress. It’s a move from responsive AI models that provide real-time information for people to interpret, to models that provide the proactive generation of insights and interpretation. This is what we call the “age of inference” where AI agents will proactively retrieve and generate data to collaboratively deliver insights and answers, not just data.
Ironwood is built to support this next phase of generative AI and its tremendous computational and communication requirements. It scales up to 9,216 liquid cooled chips linked with breakthrough Inter-Chip Interconnect (ICI) networking spanning nearly 10 MW. It is one of several new components of Google Cloud AI Hypercomputer architecture, which optimizes hardware and software together for the most demanding AI workloads. With Ironwood, developers can also leverage Google’s own Pathways software stack to reliably and easily harness the combined computing power of tens of thousands of Ironwood TPUs.
Here’s a closer look at how these innovations work together to take on the most demanding training and serving workloads with unparalleled performance, cost and power efficiency.
For Google Cloud customers, Ironwood comes in two sizes based on AI workload demands: a 256 chip configuration and a 9,216 chip configuration.
Figure 1. Improvement in the total FP8 peak flops performance relative to TPU v2, Google’s first external Cloud TPU.
Figure 2. Side by side comparison of technical specifications of the 3D torus version of Cloud TPU products including the latest generation Ironwood. FP8 peak TFlops emulated for v4 and v5p, but natively supported for Ironwood.
Figure 3. Improvement of Google’s TPU power efficiency relative to the earliest generation Cloud TPU v2. Measured by peak FP8 flops delivered per watt of thermal design power per chip package.
blog.google
Amin Vahdat
VP/GM, ML, Systems & Cloud AI
Today at Google Cloud Next 25, we’re introducing Ironwood, our seventh-generation Tensor Processing Unit (TPU) — our most performant and scalable custom AI accelerator to date, and the first designed specifically for inference. For more than a decade, TPUs have powered Google’s most demanding AI training and serving workloads, and have enabled our Cloud customers to do the same. Ironwood is our most powerful, capable and energy efficient TPU yet. And it's purpose-built to power thinking, inferential AI models at scale.
Ironwood represents a significant shift in the development of AI and the infrastructure that powers its progress. It’s a move from responsive AI models that provide real-time information for people to interpret, to models that provide the proactive generation of insights and interpretation. This is what we call the “age of inference” where AI agents will proactively retrieve and generate data to collaboratively deliver insights and answers, not just data.
Ironwood is built to support this next phase of generative AI and its tremendous computational and communication requirements. It scales up to 9,216 liquid cooled chips linked with breakthrough Inter-Chip Interconnect (ICI) networking spanning nearly 10 MW. It is one of several new components of Google Cloud AI Hypercomputer architecture, which optimizes hardware and software together for the most demanding AI workloads. With Ironwood, developers can also leverage Google’s own Pathways software stack to reliably and easily harness the combined computing power of tens of thousands of Ironwood TPUs.
Here’s a closer look at how these innovations work together to take on the most demanding training and serving workloads with unparalleled performance, cost and power efficiency.
Powering the age of inference with Ironwood
Ironwood is designed to gracefully manage the complex computation and communication demands of "thinking models," which encompass Large Language Models (LLMs), Mixture of Experts (MoEs) and advanced reasoning tasks. These models require massive parallel processing and efficient memory access. In particular, Ironwood is designed to minimize data movement and latency on chip while carrying out massive tensor manipulations. At the frontier, the computation demands of thinking models extend well beyond the capacity of any single chip. We designed Ironwood TPUs with a low-latency, high bandwidth ICI network to support coordinated, synchronous communication at full TPU pod scale.For Google Cloud customers, Ironwood comes in two sizes based on AI workload demands: a 256 chip configuration and a 9,216 chip configuration.
- When scaled to 9,216 chips per pod for a total of 42.5 Exaflops, Ironwood supports more than 24x the compute power of the world’s largest supercomputer – El Capitan – which offers just 1.7 Exaflops per pod. Ironwood delivers the massive parallel processing power necessary for the most demanding AI workloads, such as super large size dense LLM or MoE models with thinking capabilities for training and inference. Each individual chip boasts peak compute of 4,614 TFLOPs. This represents a monumental leap in AI capability. Ironwood’s memory and network architecture ensures that the right data is always available to support peak performance at this massive scale.
- Ironwood also features an enhanced SparseCore, a specialized accelerator for processing ultra-large embeddings common in advanced ranking and recommendation workloads. Expanded SparseCore support in Ironwood allows for a wider range of workloads to be accelerated, including moving beyond the traditional AI domain to financial and scientific domains.
- Pathways, Google’s own ML runtime developed by Google DeepMind, enables efficient distributed computing across multiple TPU chips. Pathways on Google Cloud makes moving beyond a single Ironwood Pod straightforward, enabling hundreds of thousands of Ironwood chips to be composed together to rapidly advance the frontiers of gen AI computation.
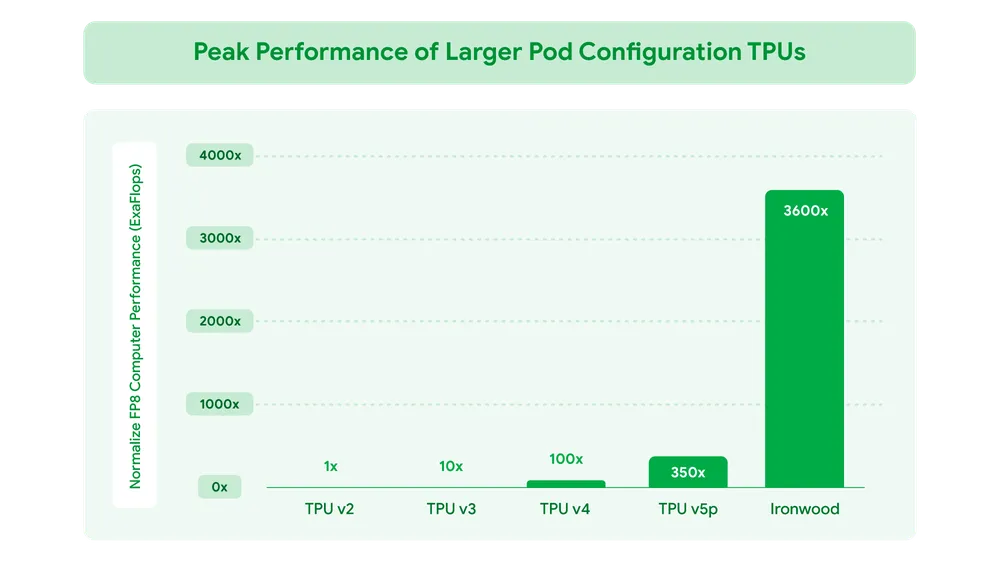
Figure 1. Improvement in the total FP8 peak flops performance relative to TPU v2, Google’s first external Cloud TPU.
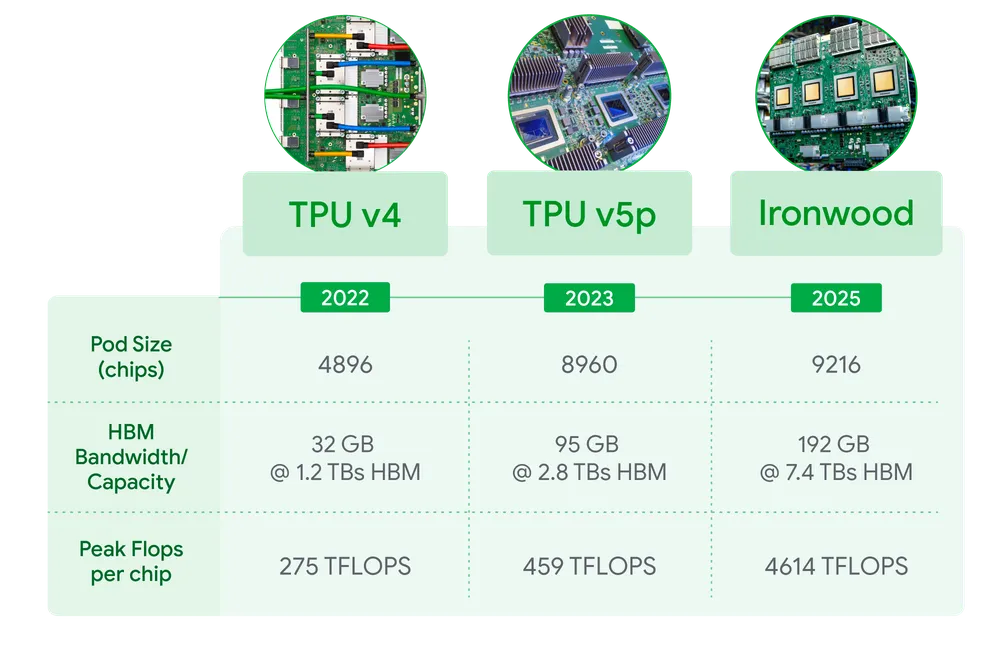
Figure 2. Side by side comparison of technical specifications of the 3D torus version of Cloud TPU products including the latest generation Ironwood. FP8 peak TFlops emulated for v4 and v5p, but natively supported for Ironwood.
Ironwood’s key features
Google Cloud is the only hyperscaler with more than a decade of experience in delivering AI compute to support cutting edge research, seamlessly integrated into planetary-scale services for billions of users every day with Gmail, Search and more. All of this expertise is at the heart of Ironwood’s capabilities. Key features include:- Significant performance gains while also focusing on power efficiency, allowing AI workloads to run more cost-effectively. Ironwood perf/watt is 2x relative to Trillium, our sixth generation TPU announced last year. At a time when available power is one of the constraints for delivering AI capabilities, we deliver significantly more capacity per watt for customer workloads. Our advanced liquid cooling solutions and optimized chip design can reliably sustain up to twice the performance of standard air cooling even under continuous, heavy AI workloads. In fact, Ironwood is nearly 30x more power efficient than our first Cloud TPU from 2018.
- Substantial increase in High Bandwidth Memory (HBM) capacity. Ironwood offers 192 GB per chip, 6x that of Trillium, which enables processing of larger models and datasets, reducing the need for frequent data transfers and improving performance.
- Dramatically improved HBM bandwidth, reaching 7.2 Tbps per chip, 4.5x of Trillium’s. This high bandwidth ensures rapid data access, crucial for memory-intensive workloads common in modern AI.
- Enhanced Inter-Chip Interconnect (ICI) bandwidth. This has been increased to 1.2 Tbps bidirectional, 1.5x of Trillium’s, enabling faster communication between chips, facilitating efficient distributed training and inference at scale.
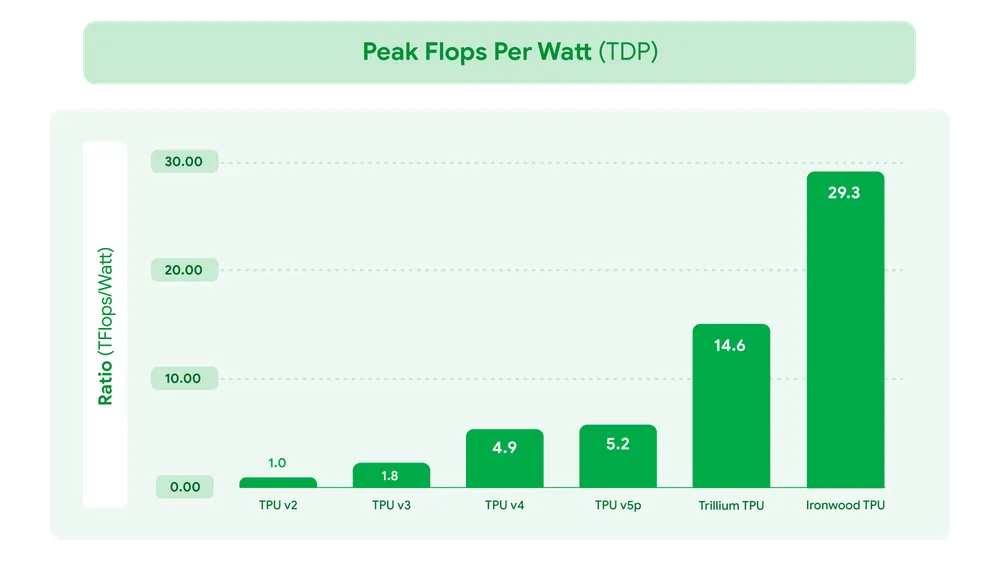
Figure 3. Improvement of Google’s TPU power efficiency relative to the earliest generation Cloud TPU v2. Measured by peak FP8 flops delivered per watt of thermal design power per chip package.
Ironwood solves the AI demands of tomorrow
Ironwood represents a unique breakthrough in the age of inference with increased computation power, memory capacity, ICI networking advancements and reliability. These breakthroughs, coupled with a nearly 2x improvement in power efficiency, mean that our most demanding customers can take on training and serving workloads with the highest performance and lowest latency, all while meeting the exponential rise in computing demand. Leading thinking models like Gemini 2.5 and the Nobel Prize winning AlphaFold all run on TPUs today, and with Ironwood we can’t wait to see what AI breakthroughs are sparked by our own developers and Google Cloud customers when it becomes available later this year.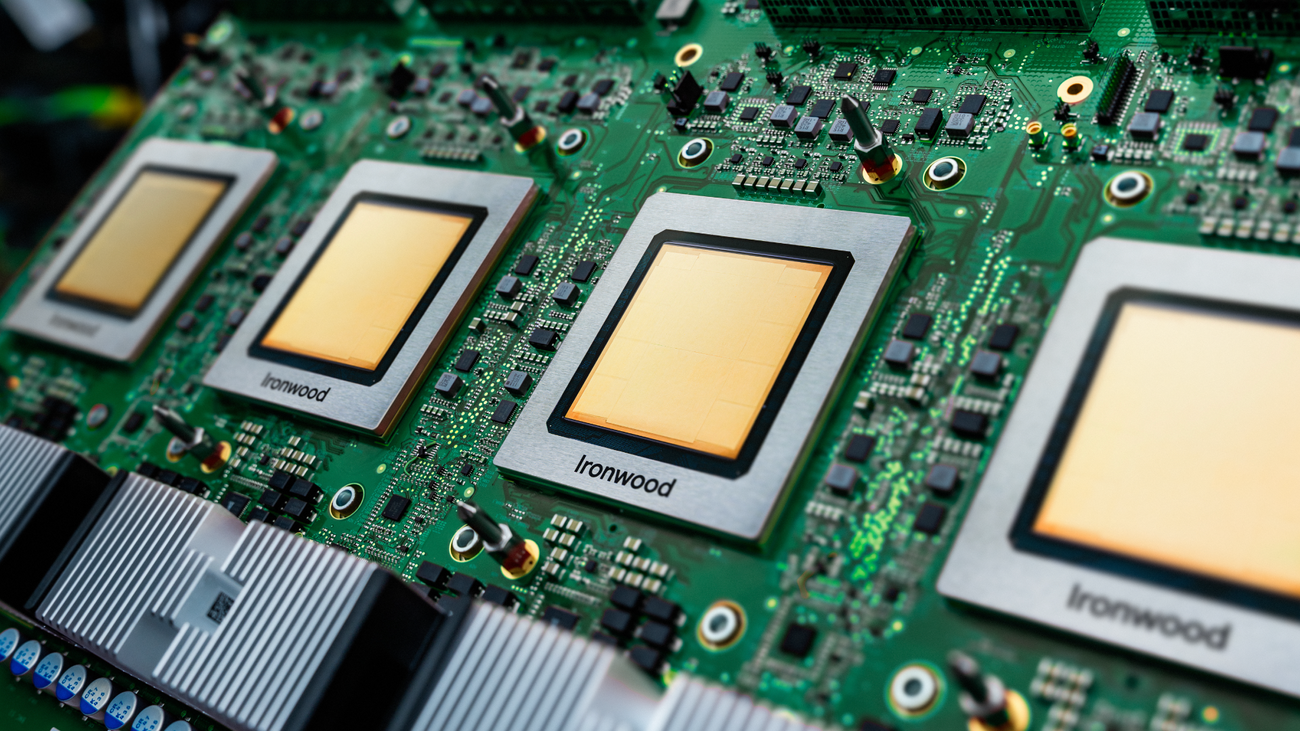
Ironwood: The first Google TPU for the age of inference
We’re introducing Ironwood, our seventh-generation Tensor Processing Unit (TPU) designed to power the age of generative AI inference.