Wanted to discuss this in light of another thread talking about how TMSC is planning to significantly increase wafer costs at 2nm.
The article indicates that cost/transistor stopped decreasing at 28nm, and is flat to rising slightly. It may actually start to rise a bit more going forward.
www.tomshardware.com
Now this does not paint the entire picture, since transistors are becoming more power efficient and there are other changes like chiplets and packaging that are leading to continued improvement, but these improvements are unlikely to push semiconductor technology further at historic rates.
I think there are two major implications here. The first is around the discussion on weather or not China can catch up on semiconductor manufacturing. I would argue that they may not need to in order to be successful. If they can drive down costs at 7nm and make their own packaging innovations, they can probably compete on a cost/performance basis in many applications.
The second implication is around AI. To a very large degree the AI industry has relied on increases in computing power to drive forward advances using larger and more sophisticated models. See below:
epochai.org
The typical AI model size, which is highly related to the compute required to train and run the models, has increased by roughly 4 orders of magnitude in the last 10 years. Thats a factor of 10,000. This is all while the cost per transistor has been flat. Anecdotally I played quite a bit with deep learning models in 2014-2016, and I could train a state of the art deep learning model on my home lab, with had 2 high end Nvidia GPUs which together cost about $2000-$3000. From what I've heard, today's deep learning models require clusters of 100+ NVidia GPUs that cost around $30k each to train. Again this is anecdotal, but a state of the art model could be trained on $3000 of hardware 10 years ago, and now a state of the art model requires at least $3,000,000 in hardware. A factor of roughly 1000.
There has still been a substantial improvement in training costs/model parameter, but I suspect room for further improvement on that metric going to be limited, and AI companies have reached the point where I don't think they can rely on building bigger models with more parameters. I could certainly be wrong though.
The article indicates that cost/transistor stopped decreasing at 28nm, and is flat to rising slightly. It may actually start to rise a bit more going forward.
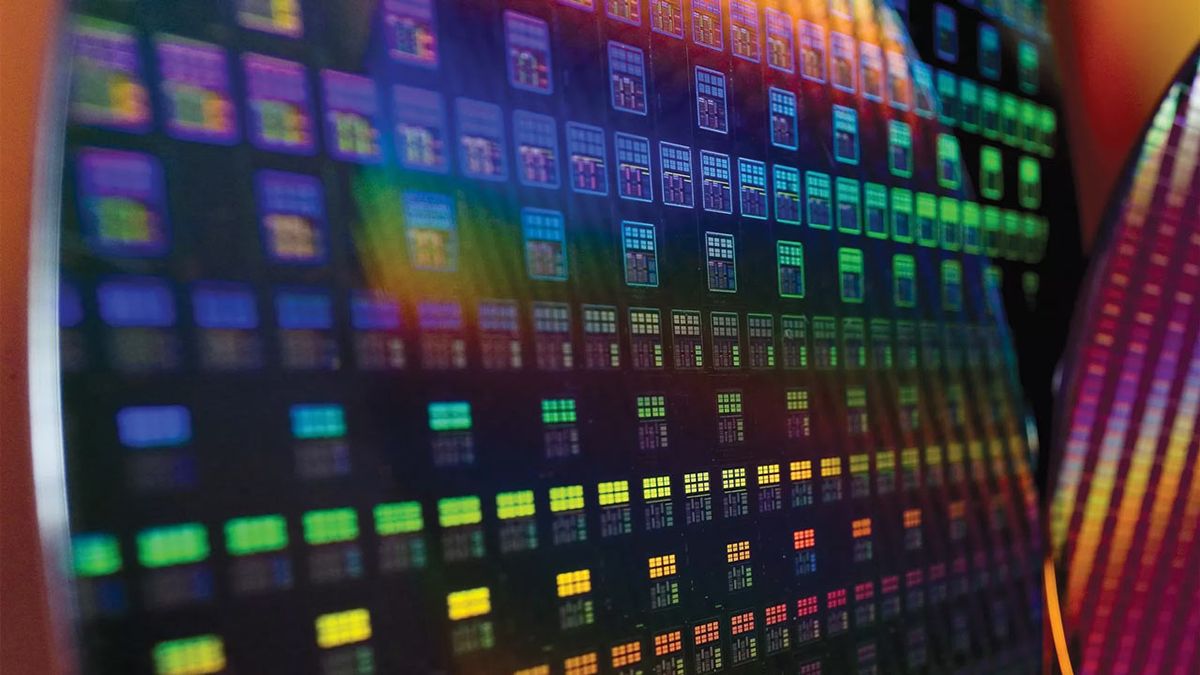
Chips aren't getting cheaper to make — the cost per transistor stopped dropping a decade ago at 28nm
Google says chips aren't getting cheaper.
Now this does not paint the entire picture, since transistors are becoming more power efficient and there are other changes like chiplets and packaging that are leading to continued improvement, but these improvements are unlikely to push semiconductor technology further at historic rates.
I think there are two major implications here. The first is around the discussion on weather or not China can catch up on semiconductor manufacturing. I would argue that they may not need to in order to be successful. If they can drive down costs at 7nm and make their own packaging innovations, they can probably compete on a cost/performance basis in many applications.
The second implication is around AI. To a very large degree the AI industry has relied on increases in computing power to drive forward advances using larger and more sophisticated models. See below:

Machine Learning Model Sizes and the Parameter Gap
The model size of notable machine learning systems has grown ten times faster than before since 2018. After 2020 growth has not been entirely continuous: there was a jump of one order of magnitude which persists until today. This is relevant for forecasting model size and thus AI capabilities.
The typical AI model size, which is highly related to the compute required to train and run the models, has increased by roughly 4 orders of magnitude in the last 10 years. Thats a factor of 10,000. This is all while the cost per transistor has been flat. Anecdotally I played quite a bit with deep learning models in 2014-2016, and I could train a state of the art deep learning model on my home lab, with had 2 high end Nvidia GPUs which together cost about $2000-$3000. From what I've heard, today's deep learning models require clusters of 100+ NVidia GPUs that cost around $30k each to train. Again this is anecdotal, but a state of the art model could be trained on $3000 of hardware 10 years ago, and now a state of the art model requires at least $3,000,000 in hardware. A factor of roughly 1000.
There has still been a substantial improvement in training costs/model parameter, but I suspect room for further improvement on that metric going to be limited, and AI companies have reached the point where I don't think they can rely on building bigger models with more parameters. I could certainly be wrong though.