The Q&A was interesting. Jensen is the best CEO in the semiconductor industry, absolutely!
Toshiya Hari -- Analyst
Hi. Good afternoon. Thank you so much for taking the question. Jensen, you executed the mass change earlier this year.
There were some reports over the weekend about some heating issues. On the back of this, we've had investors ask about your ability to execute to the road map you presented at GTC this year with Ultra coming out next year and the transition to Rubin in '26. Can you sort of speak to that? And some investors are questioning that, so if you can sort of speak to your ability to execute on time, that would be super helpful. And then a quick part B.
On supply constraints, is it a multitude of componentry that's causing this, or is it specifically HBN? Is it supply constraints? Are the supply constraints getting better? Are they worsening? Any sort of color on that would be super helpful as well. Thank you.
Jensen Huang -- President and Chief Executive Officer
Yeah. Thanks. So, let's see. Back to the first question.
Blackwell production is in full steam. In fact, as Colette mentioned earlier, we will deliver this quarter more Blackwells than we had previously estimated. And so, the supply chain team is doing an incredible job working with our supply partners to increase Blackwell, and we're going to continue to work hard to increase Blackwell through next year. It is the case that demand exceeds our supply.
And that's expected as we're in the beginnings of this generative AI revolution as we all know. And we're at the beginning of a new generation of foundation models that are able to do reasoning and able to do long thinking. And of course, one of the really exciting areas is physical AI, AI that now understands the structure of the physical world. And so, Blackwell demand is very strong.
Our execution is going well. And there's obviously a lot of engineering that we're doing across the world. You see now systems that are being stood up by Dell and CoreWeave. I think you saw systems from Oracle stood up.
You have systems from Microsoft, and they're about to preview their Grace Blackwell systems. You have systems that are at Google. And so, all of these CSPs are racing to be first. The engineering that we do with them is, as you know, rather complicated.
And the reason for that is because, although we build full stack and full infrastructure, we disaggregate all of this AI supercomputer, and we integrate it into all of the custom data centers and architectures around the world. That integration process, it's something we've done several generations now. We're very good at it but still there's still a lot of engineering that happens at this point. But as you see from all of the systems that are being stood up, Blackwell is in great shape.
And as we mentioned earlier, the supply and what we're planning to ship this quarter is greater than our previous estimates. With respect to the supply chain, there are seven different chips, seven custom chips that we built in order for us to deliver the Blackwell systems. The Blackwell systems go in air-cooled or liquid-cooled, NVLink 8 or NVLink 72 or NVLink 8, NVLink 36, NVLink 72. We have x86 or Grace.
And the integration of all of those systems into the world's data centers is nothing short of a miracle. And so, the component supply chain necessary to ramp at this scale, you have to go back and take a look at how much Blackwell we shipped last quarter, which was zero. And in terms of how much Blackwell total systems will ship this quarter, which is measured in billions, the ramp is incredible. And so almost every company in the world seems to be involved in our supply chain.
And we've got great partners, everybody from, of course, TSMC and Amphenol, the connector company, incredible company; Vertiv and SK Hynix and Micron; Spill Amcor; KYEC; and there's Foxconn and the factories that they've built; and Quanta and Wiwynn; and, gosh, Dell and HP, and Super Micro, Lenovo. And the number of companies is just really quite incredible. Quanta. And I'm sure I've missed partners that are involved in the ramping of Blackwell, which I really appreciate.
And so, anyways, I think we're in great shape with respect to the Blackwell ramp at this point. And then lastly, your question about our execution of our road map. We're on an annual road map and we're expecting to continue to execute on our annual road map. And by doing so, we increased the performance, of course, of our platform.
But it's also really important to realize that when we're able to increase performance and do so at factors at a time, we're reducing the cost of training. We're reducing the cost of inferencing. We're reducing the cost of AI so that it could be much more accessible. But the other factor that's very important to note is that when there's a data center of some fixed size and the data center always is of some fixed size.
It could be, of course, tens of megawatts in the past, and now it's -- most data centers are now 100 megawatts to several hundred megawatts, and we're planning on gigawatt data centers, it doesn't really matter how large the data centers are. The power is limited. And when you're in the power-limited data center, the best -- the highest performance per watt translates directly into the highest revenues for our partners. And so, on the one hand, our annual road map reduces costs.
But on the other hand, because our perf per watt is so good compared to anything out there, we generate for our customers the greatest possible revenues. And so, that annual rhythm is really important to us, and we have every intention of continuing to do that. And everything is on track as far as I know.
Timothy Arcuri -- Analyst
Thanks a lot. I'm wondering if you can talk about the trajectory of how Blackwell is going to ramp this year. I know Jensen, you did just talk about Blackwell being better than -- I think you had said several billions of dollars in January. It sounds like you're going to do more than that.
But I think in recent months also, you said that Blackwell crosses over Hopper in the April quarter. So, I guess I had two questions. First of all, is that still the right way to think about it, that Blackwell will cross over Hopper in April? And then Colette, you kind of talked about Blackwell bringing down gross margin to the low 70s as it ramps. So, I guess if April is the crossover, is that the worst of the pressure on gross margin? So, you're going to be kind of in the low 70s as soon as April.
Jensen Huang -- President and Chief Executive Officer
Hopper demand will continue through next year, surely the first several quarters of the next year. And meanwhile, we will ship more Blackwells next quarter than this, and we'll ship more Blackwells the quarter after that than our first quarter. And so, that kind of puts it in perspective. We are really at the beginnings of two fundamental shifts in computing that is really quite significant.
The first is moving from coding that runs on CPUs to machine learning that creates neural networks that runs on GPUs. And that fundamental shift from coding to machine learning is widespread at this point. There are no companies who are not going to do machine learning. And so, machine learning is also what enables generative AI.
And so, on the one hand, the first thing that's happening is $1 trillion worth of computing systems and data centers around the world is now being modernized for machine learning. On the other hand, secondarily, I guess, is that on top of these systems are going to be -- we're going to be creating a new type of capability called AI. And when we say generative AI, we're essentially saying that these data centers are really AI factories. They're generating something.
Just like we generate electricity, we're now going to be generating AI. And if the number of customers is large, just as the number of consumers of electricity is large, these generators are going to be running 24/7. And today, many AI services are running 24/7, just like an AI factory. And so, we're going to see this new type of system come online, and I call it an AI factory because that's really as close to what it is.
It's unlike a data center of the past. And so, these two fundamental trends are really just beginning. And so, we expect this to happen, this growth, this modernization, and the creation of a new industry to go on for several years.
Vivek Arya -- Analyst
Jensen, my main question, historically, when we have seen hardware deployment cycles, they have inevitably included some digestion along the way. When do you think we get to that phase? Or is it just too premature to discuss that because you're just at the start of Blackwell? So, how many quarters of shipments do you think is required to kind of satisfy this first wave? Can you continue to grow this into calendar '26? Just how should we be prepared to see what we have seen historically, right, a period of digestion along the way of a long-term kind of secular hardware deployment?
Jensen Huang -- President and Chief Executive Officer
The way to think through that, Vivek, is I believe that there will be no digestion until we modernize $1 trillion with the data centers. Those -- if you just look at the world's data centers, the vast majority of it is built for a time when we wrote applications by hand and we ran them on CPUs. It's just not a sensible thing to do anymore. If you have -- if every company's capex -- if they're ready to build data center tomorrow, they ought to build it for a future of machine learning and generative AI because they have plenty of old data centers.
And so, what's going to happen over the course of the next X number of years, and let's assume that over the course of four years, the world's data centers could be modernized as we grow into IT, as you know, IT continues to grow about 20%, 30% a year, let's say. But let's say by 2030, the world's data centers for computing is, call it, a couple of trillion dollars. We have to grow into that. We have to modernize the data center from coding to machine learning.
That's number one. The second part of it is generative AI. And we're now producing a new type of capability the world's never known, a new market segment that the world's never had. If you look at OpenAI, it didn't replace anything.
It's something that's completely brand new. It's, in a lot of ways as when the iPhone came, was completely brand new. It wasn't really replacing anything. And so, we're going to see more and more companies like that.
And they're going to create and generate, out of their services, essentially intelligence. Some of it would be digital artist intelligence like Runway. Some of it would be basic intelligence like OpenAI. Some of it would be legal intelligence like Harvey, digital marketing intelligence like Rider's, so on and so forth.
And the number of these companies, these -- what are they called, AI-native companies, are just in hundreds. And almost every platform shift, there was -- there were Internet companies, as you recall. There were cloud-first companies. There were mobile-first companies.
Now, they're AI natives. And so, these companies are being created because people see that there's a platform shift, and there's a brand-new opportunity to do something completely new. And so, my sense is that we're going to continue to build out to modernize IT, modernize computing, number one; and then number two, create these AI factories that are going to be for a new industry for the production of artificial intelligence.
Joseph Moore -- Analyst
Great. Thank you. I wonder if you could talk a little bit about what you're seeing in the inference market. You've talked about Strawberry and some of the ramifications of longer scaling influence projects.
But you've also talked about the possibility that as some of these Hopper clusters age, that you could use some of the Hopper chips for inference. So, I guess do you expect inference to outgrow training in the next kind of 12 months time frame? And just generally, your thoughts there.
Jensen Huang -- President and Chief Executive Officer
Our hopes and dreams is that someday, the world does a ton of inference. And that's when AI has really exceeded is when every single company is doing inference inside their companies for the marketing department and forecasting department and supply chain group and their legal department and engineering, of course, and coding of course. And so, we hope that every company is doing inference 24/7 and that there will be a whole bunch of AI native start-ups, thousands of AI native start-ups that are generating tokens and generating AI. And every aspect of your computer experience from using Outlook to PowerPointing or when you're sitting there with Excel, you're constantly generating tokens.
And every time you read a PDF, open a PDF, it generated a whole bunch of tokens. One of my favorite applications is NotebookLM, this Google application that came out. I used the living daylights out of it just because it's fun. And I put every PDF, every archived paper into it just to listen to it as well as scanning through it.
And so, I think that's the goal is to train these models so that people use it. And there's now a whole new era of AI, if you will, a whole new genre of AI called physical AI. Just those large language models understand the human language and how the thinking process, if you will. Physical AI understands the physical world.
And it understands the meaning of the structure and understands what's sensible and what's not and what could happen and what won't. And not only does it understand but it can predict, roll out a short future. That capability is incredibly valuable for industrial AI and robotics. And so, that's fired up so many AI-native companies and robotics companies and physical AI companies that you're probably hearing about.
And it's really the reason why we built Omniverse. Omniverse is -- so that we can enable these AIs to be created and learn in Omniverse and learn from synthetic data generation and reinforcement, learning physics feedback. Instead of just a human feedback, it's now physics feedback. To have these capabilities, Omniverse was created so that we can enable physical AI.
And so, that -- the goal is to generate tokens. The goal is to inference, and we're starting to see that growth happening. So, I'm super excited about that. Now, let me just say one more thing.
Inference is super hard. And the reason why inference is super hard is because you need the accuracy to be high on the one hand. You need the throughput to be high so that the cost could be as low as possible, but you also need the latency to be low. And computers that are high-throughput as well as low latency is incredibly hard to build.
And these applications have long context lengths because they want to understand. They want to be able to inference within understanding the context of what they're being asked to do. And so, the context length is growing larger and larger. On the other hand, the models are getting larger.
They're multimodality. Just the number of dimensions that inference is innovating is incredible. And this innovation rate is what makes NVIDIA's architecture so great because we -- our ecosystem is fantastic. Everybody knows that if they innovate on top of CUDA and top of NVIDIA's architecture, they can innovate more quickly and they know that everything should work.
And if something were to happen, it's probably likely their code and not ours. And so, that ability to innovate in every single direction at the same time, having a large installed base so that whatever you create could land on an NVIDIA computer and be deployed broadly all around the world in every single data center all the way out to the edge into robotic systems, that capability is really quite phenomenal.
Thank you. I'd like to turn the call back over to Jensen Huang for closing remarks.
Jensen Huang -- President and Chief Executive Officer
Thank you. The tremendous growth in our business is being fueled by two fundamental trends that are driving global adoption of NVIDIA computing. First, the computing stack is undergoing a reinvention, a platform shift from coding to machine learning, from executing code on CPUs to processing neural networks on GPUs. The $1 trillion installed base of traditional data center infrastructure is being rebuilt for Software 2.0, which applies machine learning to produce AI.
Second, the age of AI is in full steam. Generative AI is not just a new software capability but a new industry with AI factories manufacturing digital intelligence, a new industrial revolution that can create a multi-trillion-dollar AI industry. Demand for Hopper and anticipation for Blackwell, which is now in full production, are incredible for several reasons. There are more foundation model makers now than there were a year ago.
The computing scale of pretraining and post-training continues to grow exponentially. There are more AI-native start-ups than ever, and the number of successful inference services is rising. And with the introduction of ChatGPT o1, OpenAI o1, a new scaling law called test time scaling has emerged. All of these consume a great deal of computing.
AI is transforming every industry, company, and country. Enterprises are adopting agentic AI to revolutionize workflows. Over time, AI coworkers will assist employees in performing their jobs faster and better. Investments in industrial robotics are surging due to breakthroughs in physical AI, driving new training infrastructure demand as researchers train world foundation models on petabytes of video and Omniverse synthetically generated data.
The age of robotics is coming. Countries across the world recognize the fundamental AI trends we are seeing and have awakened to the importance of developing their national AI infrastructure. The age of AI is upon us, and it's large and diverse. NVIDIA's expertise, scale, and ability to deliver full stack and full infrastructure lets us serve the entire multitrillion-dollar AI and robotics opportunities ahead from every hyperscale cloud, enterprise private cloud to sovereign regional AI clouds, on-prem to industrial edge and robotics.
Thanks for joining us today, and catch up next time.
www.fool.com
Toshiya Hari -- Analyst
Hi. Good afternoon. Thank you so much for taking the question. Jensen, you executed the mass change earlier this year.
There were some reports over the weekend about some heating issues. On the back of this, we've had investors ask about your ability to execute to the road map you presented at GTC this year with Ultra coming out next year and the transition to Rubin in '26. Can you sort of speak to that? And some investors are questioning that, so if you can sort of speak to your ability to execute on time, that would be super helpful. And then a quick part B.
On supply constraints, is it a multitude of componentry that's causing this, or is it specifically HBN? Is it supply constraints? Are the supply constraints getting better? Are they worsening? Any sort of color on that would be super helpful as well. Thank you.
Jensen Huang -- President and Chief Executive Officer
Yeah. Thanks. So, let's see. Back to the first question.
Blackwell production is in full steam. In fact, as Colette mentioned earlier, we will deliver this quarter more Blackwells than we had previously estimated. And so, the supply chain team is doing an incredible job working with our supply partners to increase Blackwell, and we're going to continue to work hard to increase Blackwell through next year. It is the case that demand exceeds our supply.
And that's expected as we're in the beginnings of this generative AI revolution as we all know. And we're at the beginning of a new generation of foundation models that are able to do reasoning and able to do long thinking. And of course, one of the really exciting areas is physical AI, AI that now understands the structure of the physical world. And so, Blackwell demand is very strong.
Our execution is going well. And there's obviously a lot of engineering that we're doing across the world. You see now systems that are being stood up by Dell and CoreWeave. I think you saw systems from Oracle stood up.
You have systems from Microsoft, and they're about to preview their Grace Blackwell systems. You have systems that are at Google. And so, all of these CSPs are racing to be first. The engineering that we do with them is, as you know, rather complicated.
And the reason for that is because, although we build full stack and full infrastructure, we disaggregate all of this AI supercomputer, and we integrate it into all of the custom data centers and architectures around the world. That integration process, it's something we've done several generations now. We're very good at it but still there's still a lot of engineering that happens at this point. But as you see from all of the systems that are being stood up, Blackwell is in great shape.
And as we mentioned earlier, the supply and what we're planning to ship this quarter is greater than our previous estimates. With respect to the supply chain, there are seven different chips, seven custom chips that we built in order for us to deliver the Blackwell systems. The Blackwell systems go in air-cooled or liquid-cooled, NVLink 8 or NVLink 72 or NVLink 8, NVLink 36, NVLink 72. We have x86 or Grace.
And the integration of all of those systems into the world's data centers is nothing short of a miracle. And so, the component supply chain necessary to ramp at this scale, you have to go back and take a look at how much Blackwell we shipped last quarter, which was zero. And in terms of how much Blackwell total systems will ship this quarter, which is measured in billions, the ramp is incredible. And so almost every company in the world seems to be involved in our supply chain.
And we've got great partners, everybody from, of course, TSMC and Amphenol, the connector company, incredible company; Vertiv and SK Hynix and Micron; Spill Amcor; KYEC; and there's Foxconn and the factories that they've built; and Quanta and Wiwynn; and, gosh, Dell and HP, and Super Micro, Lenovo. And the number of companies is just really quite incredible. Quanta. And I'm sure I've missed partners that are involved in the ramping of Blackwell, which I really appreciate.
And so, anyways, I think we're in great shape with respect to the Blackwell ramp at this point. And then lastly, your question about our execution of our road map. We're on an annual road map and we're expecting to continue to execute on our annual road map. And by doing so, we increased the performance, of course, of our platform.
But it's also really important to realize that when we're able to increase performance and do so at factors at a time, we're reducing the cost of training. We're reducing the cost of inferencing. We're reducing the cost of AI so that it could be much more accessible. But the other factor that's very important to note is that when there's a data center of some fixed size and the data center always is of some fixed size.
It could be, of course, tens of megawatts in the past, and now it's -- most data centers are now 100 megawatts to several hundred megawatts, and we're planning on gigawatt data centers, it doesn't really matter how large the data centers are. The power is limited. And when you're in the power-limited data center, the best -- the highest performance per watt translates directly into the highest revenues for our partners. And so, on the one hand, our annual road map reduces costs.
But on the other hand, because our perf per watt is so good compared to anything out there, we generate for our customers the greatest possible revenues. And so, that annual rhythm is really important to us, and we have every intention of continuing to do that. And everything is on track as far as I know.
Timothy Arcuri -- Analyst
Thanks a lot. I'm wondering if you can talk about the trajectory of how Blackwell is going to ramp this year. I know Jensen, you did just talk about Blackwell being better than -- I think you had said several billions of dollars in January. It sounds like you're going to do more than that.
But I think in recent months also, you said that Blackwell crosses over Hopper in the April quarter. So, I guess I had two questions. First of all, is that still the right way to think about it, that Blackwell will cross over Hopper in April? And then Colette, you kind of talked about Blackwell bringing down gross margin to the low 70s as it ramps. So, I guess if April is the crossover, is that the worst of the pressure on gross margin? So, you're going to be kind of in the low 70s as soon as April.
Jensen Huang -- President and Chief Executive Officer
Hopper demand will continue through next year, surely the first several quarters of the next year. And meanwhile, we will ship more Blackwells next quarter than this, and we'll ship more Blackwells the quarter after that than our first quarter. And so, that kind of puts it in perspective. We are really at the beginnings of two fundamental shifts in computing that is really quite significant.
The first is moving from coding that runs on CPUs to machine learning that creates neural networks that runs on GPUs. And that fundamental shift from coding to machine learning is widespread at this point. There are no companies who are not going to do machine learning. And so, machine learning is also what enables generative AI.
And so, on the one hand, the first thing that's happening is $1 trillion worth of computing systems and data centers around the world is now being modernized for machine learning. On the other hand, secondarily, I guess, is that on top of these systems are going to be -- we're going to be creating a new type of capability called AI. And when we say generative AI, we're essentially saying that these data centers are really AI factories. They're generating something.
Just like we generate electricity, we're now going to be generating AI. And if the number of customers is large, just as the number of consumers of electricity is large, these generators are going to be running 24/7. And today, many AI services are running 24/7, just like an AI factory. And so, we're going to see this new type of system come online, and I call it an AI factory because that's really as close to what it is.
It's unlike a data center of the past. And so, these two fundamental trends are really just beginning. And so, we expect this to happen, this growth, this modernization, and the creation of a new industry to go on for several years.
Vivek Arya -- Analyst
Jensen, my main question, historically, when we have seen hardware deployment cycles, they have inevitably included some digestion along the way. When do you think we get to that phase? Or is it just too premature to discuss that because you're just at the start of Blackwell? So, how many quarters of shipments do you think is required to kind of satisfy this first wave? Can you continue to grow this into calendar '26? Just how should we be prepared to see what we have seen historically, right, a period of digestion along the way of a long-term kind of secular hardware deployment?
Jensen Huang -- President and Chief Executive Officer
The way to think through that, Vivek, is I believe that there will be no digestion until we modernize $1 trillion with the data centers. Those -- if you just look at the world's data centers, the vast majority of it is built for a time when we wrote applications by hand and we ran them on CPUs. It's just not a sensible thing to do anymore. If you have -- if every company's capex -- if they're ready to build data center tomorrow, they ought to build it for a future of machine learning and generative AI because they have plenty of old data centers.
And so, what's going to happen over the course of the next X number of years, and let's assume that over the course of four years, the world's data centers could be modernized as we grow into IT, as you know, IT continues to grow about 20%, 30% a year, let's say. But let's say by 2030, the world's data centers for computing is, call it, a couple of trillion dollars. We have to grow into that. We have to modernize the data center from coding to machine learning.
That's number one. The second part of it is generative AI. And we're now producing a new type of capability the world's never known, a new market segment that the world's never had. If you look at OpenAI, it didn't replace anything.
It's something that's completely brand new. It's, in a lot of ways as when the iPhone came, was completely brand new. It wasn't really replacing anything. And so, we're going to see more and more companies like that.
And they're going to create and generate, out of their services, essentially intelligence. Some of it would be digital artist intelligence like Runway. Some of it would be basic intelligence like OpenAI. Some of it would be legal intelligence like Harvey, digital marketing intelligence like Rider's, so on and so forth.
And the number of these companies, these -- what are they called, AI-native companies, are just in hundreds. And almost every platform shift, there was -- there were Internet companies, as you recall. There were cloud-first companies. There were mobile-first companies.
Now, they're AI natives. And so, these companies are being created because people see that there's a platform shift, and there's a brand-new opportunity to do something completely new. And so, my sense is that we're going to continue to build out to modernize IT, modernize computing, number one; and then number two, create these AI factories that are going to be for a new industry for the production of artificial intelligence.
Joseph Moore -- Analyst
Great. Thank you. I wonder if you could talk a little bit about what you're seeing in the inference market. You've talked about Strawberry and some of the ramifications of longer scaling influence projects.
But you've also talked about the possibility that as some of these Hopper clusters age, that you could use some of the Hopper chips for inference. So, I guess do you expect inference to outgrow training in the next kind of 12 months time frame? And just generally, your thoughts there.
Jensen Huang -- President and Chief Executive Officer
Our hopes and dreams is that someday, the world does a ton of inference. And that's when AI has really exceeded is when every single company is doing inference inside their companies for the marketing department and forecasting department and supply chain group and their legal department and engineering, of course, and coding of course. And so, we hope that every company is doing inference 24/7 and that there will be a whole bunch of AI native start-ups, thousands of AI native start-ups that are generating tokens and generating AI. And every aspect of your computer experience from using Outlook to PowerPointing or when you're sitting there with Excel, you're constantly generating tokens.
And every time you read a PDF, open a PDF, it generated a whole bunch of tokens. One of my favorite applications is NotebookLM, this Google application that came out. I used the living daylights out of it just because it's fun. And I put every PDF, every archived paper into it just to listen to it as well as scanning through it.
And so, I think that's the goal is to train these models so that people use it. And there's now a whole new era of AI, if you will, a whole new genre of AI called physical AI. Just those large language models understand the human language and how the thinking process, if you will. Physical AI understands the physical world.
And it understands the meaning of the structure and understands what's sensible and what's not and what could happen and what won't. And not only does it understand but it can predict, roll out a short future. That capability is incredibly valuable for industrial AI and robotics. And so, that's fired up so many AI-native companies and robotics companies and physical AI companies that you're probably hearing about.
And it's really the reason why we built Omniverse. Omniverse is -- so that we can enable these AIs to be created and learn in Omniverse and learn from synthetic data generation and reinforcement, learning physics feedback. Instead of just a human feedback, it's now physics feedback. To have these capabilities, Omniverse was created so that we can enable physical AI.
And so, that -- the goal is to generate tokens. The goal is to inference, and we're starting to see that growth happening. So, I'm super excited about that. Now, let me just say one more thing.
Inference is super hard. And the reason why inference is super hard is because you need the accuracy to be high on the one hand. You need the throughput to be high so that the cost could be as low as possible, but you also need the latency to be low. And computers that are high-throughput as well as low latency is incredibly hard to build.
And these applications have long context lengths because they want to understand. They want to be able to inference within understanding the context of what they're being asked to do. And so, the context length is growing larger and larger. On the other hand, the models are getting larger.
They're multimodality. Just the number of dimensions that inference is innovating is incredible. And this innovation rate is what makes NVIDIA's architecture so great because we -- our ecosystem is fantastic. Everybody knows that if they innovate on top of CUDA and top of NVIDIA's architecture, they can innovate more quickly and they know that everything should work.
And if something were to happen, it's probably likely their code and not ours. And so, that ability to innovate in every single direction at the same time, having a large installed base so that whatever you create could land on an NVIDIA computer and be deployed broadly all around the world in every single data center all the way out to the edge into robotic systems, that capability is really quite phenomenal.
Thank you. I'd like to turn the call back over to Jensen Huang for closing remarks.
Jensen Huang -- President and Chief Executive Officer
Thank you. The tremendous growth in our business is being fueled by two fundamental trends that are driving global adoption of NVIDIA computing. First, the computing stack is undergoing a reinvention, a platform shift from coding to machine learning, from executing code on CPUs to processing neural networks on GPUs. The $1 trillion installed base of traditional data center infrastructure is being rebuilt for Software 2.0, which applies machine learning to produce AI.
Second, the age of AI is in full steam. Generative AI is not just a new software capability but a new industry with AI factories manufacturing digital intelligence, a new industrial revolution that can create a multi-trillion-dollar AI industry. Demand for Hopper and anticipation for Blackwell, which is now in full production, are incredible for several reasons. There are more foundation model makers now than there were a year ago.
The computing scale of pretraining and post-training continues to grow exponentially. There are more AI-native start-ups than ever, and the number of successful inference services is rising. And with the introduction of ChatGPT o1, OpenAI o1, a new scaling law called test time scaling has emerged. All of these consume a great deal of computing.
AI is transforming every industry, company, and country. Enterprises are adopting agentic AI to revolutionize workflows. Over time, AI coworkers will assist employees in performing their jobs faster and better. Investments in industrial robotics are surging due to breakthroughs in physical AI, driving new training infrastructure demand as researchers train world foundation models on petabytes of video and Omniverse synthetically generated data.
The age of robotics is coming. Countries across the world recognize the fundamental AI trends we are seeing and have awakened to the importance of developing their national AI infrastructure. The age of AI is upon us, and it's large and diverse. NVIDIA's expertise, scale, and ability to deliver full stack and full infrastructure lets us serve the entire multitrillion-dollar AI and robotics opportunities ahead from every hyperscale cloud, enterprise private cloud to sovereign regional AI clouds, on-prem to industrial edge and robotics.
Thanks for joining us today, and catch up next time.
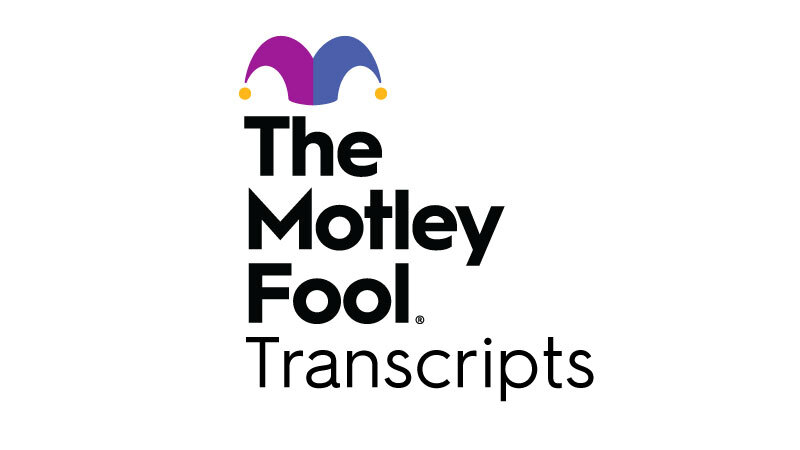
Nvidia (NVDA) Q3 2025 Earnings Call Transcript | The Motley Fool
NVDA earnings call for the period ending September 30, 2024.
