I attended Hot Chips virtually ( mostly from my boat ) and another SemiWiki blogger attended live. Anastasia also attended live and did a nice little summary that I agree with completely. AI has definitely launched in chip design and the infrastructure build is ramping up. My concern is that the cost of the infrastructure build by far outspends the current AI revenue models. It seems that AI egos are in play here and while there will be AI winners, the AI losers will out number them exponentially. AI is a risky bet but a very popular one on Wall Street so it will be fun to watch.
The Hot Chips 2024 conference took place last week at Stanford. I had the pleasure of attending in person, and in addition to the amazing program, the conversations with people between sessions were very insightful. Here are my highlights:
#1 Accelerating AI
AI was the central focus of Hot Chips 2024. More than 15 AI chips were presented at the conference, including many innovations around AI accelerators and infrastructure.

During the keynote, Trevor Cai from OpenAI discussed how scaling up has consistently yielded better and more useful AI models, the trend that is expected to continue. Here are four takeaways from the keynote:

An interesting point is that despite the heavy push for performance, it is only one of the four main factors. Another significant challenge is scaling and building a robust infrastructure to support it.
#2 Memory bottleneck
Generative AI applications are constrained by memory, so addressing the memory bottleneck is essential for scaling AI models. Nvidia, AMD, SambaNova, Meta, Tenstorrent, and Cerebras presented different approaches to overcoming it. Among them, Cerebras' architecture stands out.

Cerebras' Wafer-Scale Engine integrates 44GB of SRAM directly on the chip, enabling very fast memory access times. This is one of the reasons they’ve achieved remarkable inference speeds:
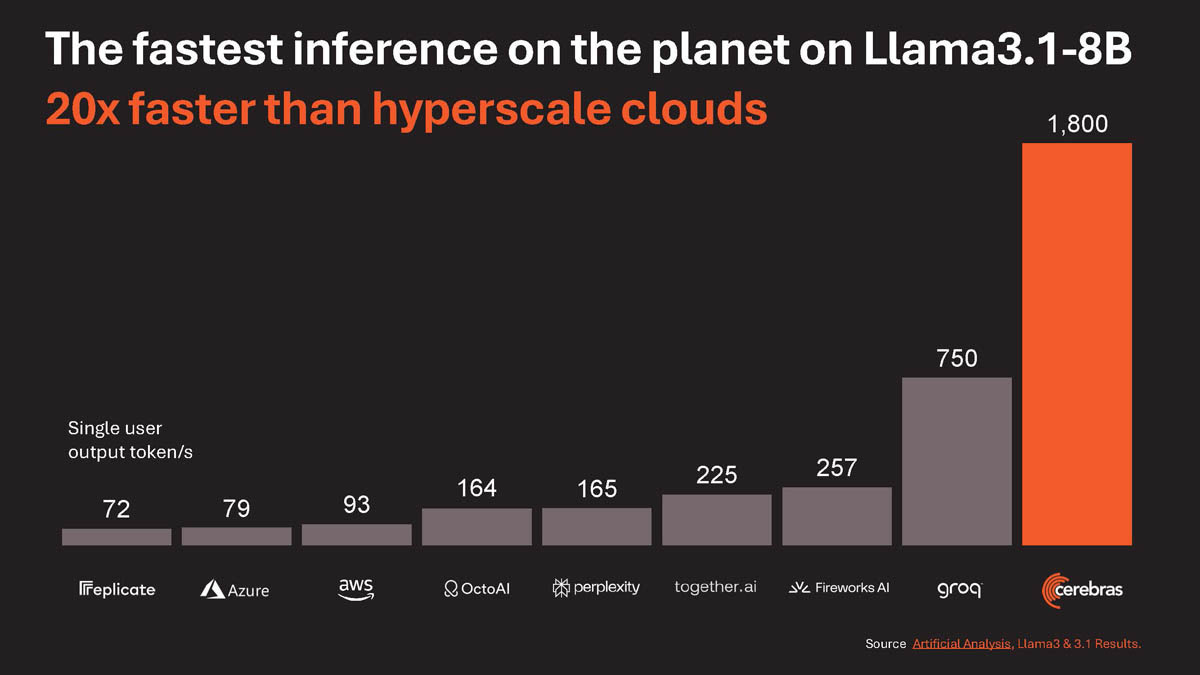
#3 AI-Assisted Chip Design
The title of the session was "Will AI Elevate or Replace Hardware Engineers?” The answer is: both. Chipmakers are adopting AI tools in their processes to boost the efficiency and automate some time-consuming tasks. AI agents are already being used for circuit design and layout optimization, fixing DRC violations, and timing closure. In one of the sessions, NVIDIA discussed how LLMs assist their engineers with answering questions, debugging design problems and more. They also use AI agents for timing report analysis, layout optimization, and code generation.
Stelios Diamantidis from Synopsys highlighted how AI is transforming electronic design automation (EDA) tools. As of today, Synopsys AI-based tools have been used to optimize performance, power, and area across more than 1,000 chip tapeouts. In some cases, they have helped shorten the chip development cycle from two years to one year.
anastasiintech.substack.com
The Hot Chips 2024 conference took place last week at Stanford. I had the pleasure of attending in person, and in addition to the amazing program, the conversations with people between sessions were very insightful. Here are my highlights:
#1 Accelerating AI
AI was the central focus of Hot Chips 2024. More than 15 AI chips were presented at the conference, including many innovations around AI accelerators and infrastructure.

During the keynote, Trevor Cai from OpenAI discussed how scaling up has consistently yielded better and more useful AI models, the trend that is expected to continue. Here are four takeaways from the keynote:

An interesting point is that despite the heavy push for performance, it is only one of the four main factors. Another significant challenge is scaling and building a robust infrastructure to support it.
#2 Memory bottleneck
Generative AI applications are constrained by memory, so addressing the memory bottleneck is essential for scaling AI models. Nvidia, AMD, SambaNova, Meta, Tenstorrent, and Cerebras presented different approaches to overcoming it. Among them, Cerebras' architecture stands out.

Cerebras' Wafer-Scale Engine integrates 44GB of SRAM directly on the chip, enabling very fast memory access times. This is one of the reasons they’ve achieved remarkable inference speeds:
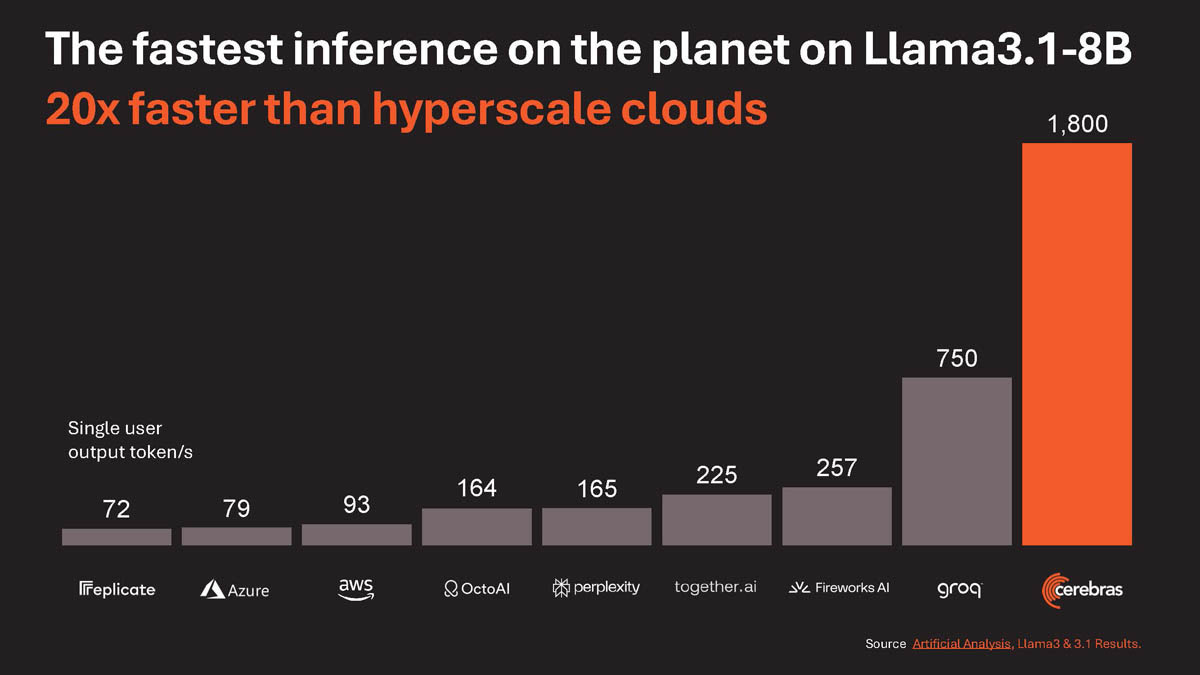
#3 AI-Assisted Chip Design
The title of the session was "Will AI Elevate or Replace Hardware Engineers?” The answer is: both. Chipmakers are adopting AI tools in their processes to boost the efficiency and automate some time-consuming tasks. AI agents are already being used for circuit design and layout optimization, fixing DRC violations, and timing closure. In one of the sessions, NVIDIA discussed how LLMs assist their engineers with answering questions, debugging design problems and more. They also use AI agents for timing report analysis, layout optimization, and code generation.
Stelios Diamantidis from Synopsys highlighted how AI is transforming electronic design automation (EDA) tools. As of today, Synopsys AI-based tools have been used to optimize performance, power, and area across more than 1,000 chip tapeouts. In some cases, they have helped shorten the chip development cycle from two years to one year.
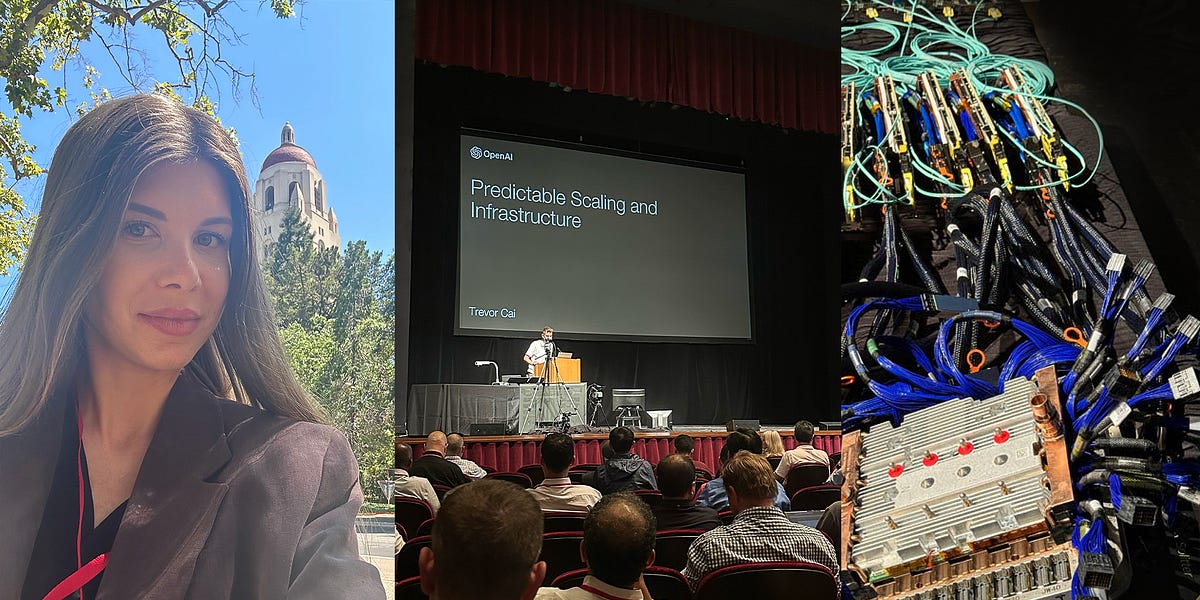
Hot Chips 2024: The Hottest Highlights
The Hot Chips 2024 conference took place last week at Stanford.